Successful AI Ethics & Governance at Scale: Bridging The Interpretation Gap
Principles that generalize require professionals who specialize
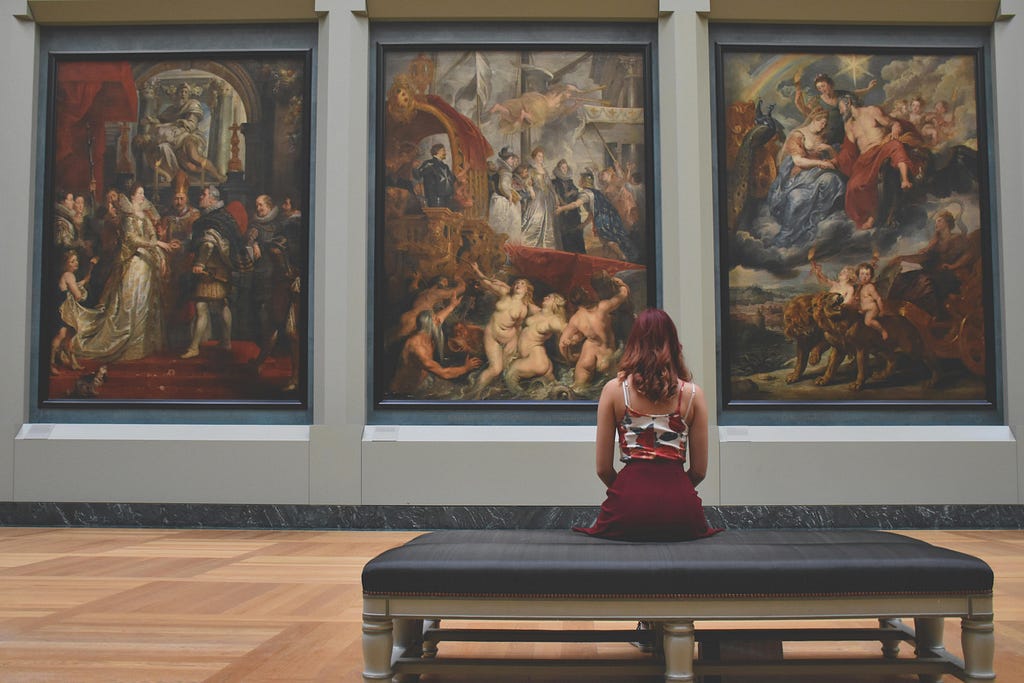
AI ethics and governance has become a noisy space.
At last count, the OECD tracker counts over 1,800 national-level documents on initiatives, policies, frameworks, and strategies as of September, 2024 (and there seems to be consultants and influencers opining on every one).
However, as Mittelstadt (2021) succinctly puts in a way that only academic understatement can, principles alone cannot guarantee ethical AI.
Despite the abundance of high-level guidance, there remains a notable gap between policy and real-world implementation. But why is this the case, and how should data science and AI leaders think about it?
In this series, I aim to advance the maturity of practical AI ethics and governance within organizations by breaking down this gap into three components, and draw from research and real world experience to propose strategies and structures that have worked in implementing AI ethics and governance capabilities at scale.
The first gap I cover is the interpretation gap, which arises from the challenge of applying principles expressed in vague language such as ‘human centricity’ and ‘fairness’ to the diverse range of real-world AI applications that are both built and purchased. Yet accounting for the nuances and complexities of each specific application is critical for effective implementation.
The Interpretation Gap in AI Ethics and Governance: Bridging Principles and Real World Practice
A significant challenge in the realm of AI ethics and governance is the interpretation and application of broadly defined ethical principles to diverse AI applications.
AI ethics principles themselves are not the primary issue. The problem is consensus on principles but divergence in practical interpretation.
There is a strong level of harmonization and consensus on the principles that responsible and trusted AI systems should have. Jobin et al. (2019) tabulated principles that appear across the global AI governance landscape and found that principles such as transparency, justice & fairness, non-maleficence, responsibility and privacy appear in over half of all published AI governance documents. Similarly a study by Fjeld et al. (2020) the following year uncovered a growing consensus towards thematic trends which she dubbed an emerging “normative core” of AI ethics principles.
However, directing AI scientists and engineers to adhere to these principles in isolation without some form of assistance to interpret them is often unhelpful.
This is driven by three reasons:
1. The Language of AI Ethics Principles are Ambiguous by Design
The first reason is that the foundational principles of AI ethics, such as human centricity and fairness, are often articulated in a manner that is conceptually broad and open to interpretation. This vagueness is needed for generalization — principles should apply across all AI use cases — but the side effect is it results in plenty of competing interpretations.
AI teams are often multi-disciplinary, with each group having rich and distinct professional cultures. Each also has varying levels of familiarity with issues related to AI ethics. Add to that mix that most practitioners not yet possessing formal education in AI ethics and governance, and we have a recipe for misinterpretation all round.
To compound the issue, depending on one’s area of focus, terms may also carry different meanings — for example, ‘transparency’ from the perspective of a data scientist training a model may carry the connotation of the inner workings of a model, while ‘transparency’ from an operations team may be conflated with ‘observability’ of the performance of the system in terms of uptime and usage, and ‘transparency’ from a privacy perspective may have to do more with ‘disclosure’ of data used in relation to the AI system.
2. AI Applications are Diverse & Complex
The second reason is that the AI principles must cover a wide variety of AI applications that span a multitude of domains. But possesses unique characteristics and have a unique view of what a ‘good’ AI model looks like.
For example, ‘accuracy’ alone is highly task dependent. An autonomous manufacturing process using video cameras and computer vision models for segmentation may prioritize precision, with metrics such as Intersection of Union (IoU) that suit the imaging modality. Chatbots powered by large language models would likely instead focus on linguistic metrics such as Massive Multitask Language Understanding (MMLU) and benchmark against toxicity and bias datasets.
An added layer of complexity exists within AI use cases. Taking the example of marketing recommendation systems, Zhang et al. (2021) in his review of state of the art approaches at the time discusses no less than six AI techniques that are used within recommender systems to enhance them, which cross both structured and unstructured data.
Thus applying a given ethical principle, such as fairness, requires nuanced understanding at the level of both the use case and the underlying methods that were implemented it in order to be contextually relevant.
3. Specialist Talent is Needed, but in Short Supply
The third reason is the need for specialist interpretation skills. The need to assist AI teams to interpret responsible AI principles in generic language, apply it appropriately to distinct AI systems, and do so with a reasonable understanding of its technical underpinnings and limitations is difficult and requires a rare combination of skills.
The implication of applying general AI ethics principles to unique AI systems is the need for specialists in AI ethics
This tends to either require specialist talent, or multiple teams working in unison, often around a complex process that operationalizes a multidisciplinary approach, integrating insights from AI developers, ethicists, sociologists, and industry-specific experts. This requires a maturity in sourcing and accessing talent which many organizations are still working to reach.
Conclusion
The interpretation gap in AI ethics and governance presents significant challenges due to ambiguity in applying general principles to diverse and complex AI applications, compounded by a lack of talent to bridge them.
However, bridging the interpretation gap is vital for AI to be effective, and doing so offers an opportunity to develop the ability to take a nuanced and fit-for-purpose approach to managing AI E&G.
References
a. Mittelstadt, B. Principles alone cannot guarantee ethical AI. Nat Mach Intell 1, 501–507 (2019). https://doi.org/10.1038/s42256-019-0114-4
b. Fjeld, J., Achten, N., Hilligoss, H., Nagy, A., & Srikumar, M. (2020). Principled AI: Mapping Consensus in Ethical and Rights-based Approaches to Principles for AI. SSRN Electronic Journal.
c. Jobin, A., Ienca, M., & Vayena, E. (2019). The global landscape of AI ethics guidelines. Nature Machine Intelligence, 1(9), 389–399.
d. Zhang, Q., Lu, J. & Jin, Y. AI in recommender systems. Complex Intell. Syst. 7, 439–457 (2021). https://doi.org/10.1007/s40747-020-00212-w
Successful AI Ethics & Governance at Scale: Bridging The Interpretation Gap was originally published in Towards Data Science on Medium, where people are continuing the conversation by highlighting and responding to this story.
from AI in Towards Data Science on Medium https://ift.tt/tB02y6N
via IFTTT