Four Career-Savers Data Scientists Should Incorporate into Their Work
You might damage your data science career progress without even realising it — but avoiding that fate isn’t too difficult
You could be self-sabotaging your data science career without even realising it. In this article, I want to discuss the four biggest career-killers I have seen, some of which I’ve also fallen victim to.
Think Business Impact, Not Technical
Most entry-level and junior data scientists focus too much on the technical details. I did this too.
I was more interested in how I could use a neural network to solve X or how XGBoost could model Y. Having this passion and excitement is great; it means you are interested in the field and you are happy to learn.
However, it’s pretty misplaced.
Your job is to improve the way the business functions and operates. Fundamentally, you are there to generate more money for the business. It may be a bit reductive, but it is true if we are brutally honest.
I remember my line manager early on in my career telling me to “focus on impact.” At the time, I thought this was just something that sounded great but was not actually tangible.
In reality, “focus on impact” really means solving business problems. What in your domain is the business or stakeholders struggling with? How can you make their lives easier?
These are the questions you should ask first before thinking of technical solutions. Ideally, you would start simple to produce immediate “impact” and then iterate from there.
Data Scientists who focus on impact will grow and get promoted quickly because they generate tangible value for the business. It doesn’t matter if you used linear regression or an RNN; if you brought in millions of pounds in revenue, no one is going to complain.
What you work on matters more than how hard you work or what complex technical things you implement. So, choose your work and projects wisely, and your career growth will accelerate.
Long Term Vision
I recently wrote in my newsletter that people should think more. It may sound philosophical and “woo-woo,” but how often do you just sit and think without distractions?
Call me sad, but I think about my career and professional growth a lot. I ask myself questions like
- What do you want to do in 1, 5 and 10 years?
- What do you actually want to do for work?
- What kind of company do you want to work at?
- Where do you want to work?
- Can I see myself doing this forever?
- What would make my job better?
- What’s my favourite part of the job?
These are essentially journaling prompts, but I use them as thinking prompts. Allowing yourself to explore and be honest with yourself really helps give you internal direction, which will eventually manifest in the real world.
Let me give you an example. In my scenario, I thought deeply about what I like most about being a data scientist and what I actually want to do going forward. For me, the best part is implementing your algorithm into production and generating tangible business value.
This is a small part of data scientists’ workflow, but another job does this all the time. It’s called a machine learning engineer, and it became clear in my head that’s what I want to do.
So, over the last few months, I have learned more about software engineering, taken a data structures and algorithms course, and am due to start a machine learning engineer role in a couple of months.
This is not to boast but to demonstrate how answering these questions gave me clarity on where I want to go. It gave me a long-term vision for my career instead of being a passenger and just going with the flow.
It’s important to be forward-looking because you may want to slightly change your role, the team you work in, or the specialism in which you want to be an expert.
I suggest sitting down and answering the questions above honestly to yourself, whether in your head or, even better, writing them down. This will give you direction in your career, which is more important than you know.
Save & Invest
Maybe this isn’t just data science advice but general work and life advice. Jobs can be fickle, and redundancies are not abnormal in today’s economic climate.
So, no matter who you are, make sure you save and preferably invest for any potential rainy day ahead.
From reading the “Psychology of Money,” I learned that people think about saving and investing differently. So, just save and invest your money how you want to that allows you to sleep comfortably at night.
There are different ways of doing this, such as investing in the stock market, interest rate accounts, and even riskier assets like crypto. I have linked an article and flow chart below that dives deeper into these topics so you can find the right one for you.
The Flowchart - UKPersonalFinance Wiki
Needless to say, this is not financial advice, and I am not a financial advisor.
The main thing you should take away is to think more about what you are doing with your money.
Explore & Learn
I see this final part way too often, and it may be a bit controversial, but I don’t think moving up the ranks really quickly is the best idea.
Hear me out.
I have seen people skyrocket into senior positions, which is great, and huge congratulations to them. However, you now box yourself in because you are likely really good in one area at one particular company with a niche skillset.
If you want to move or even get made redundant, it will be difficult for you to find a position where you are compensated and ranked equal to your current role.
You haven’t allowed yourself to explore and learn complementary skills and knowledge to make you a flexible and well-rounded data scientist.
I recommend having T-shaped skills, where you know the fundamentals very well and know roughly three areas to a pretty good depth to keep you balanced.
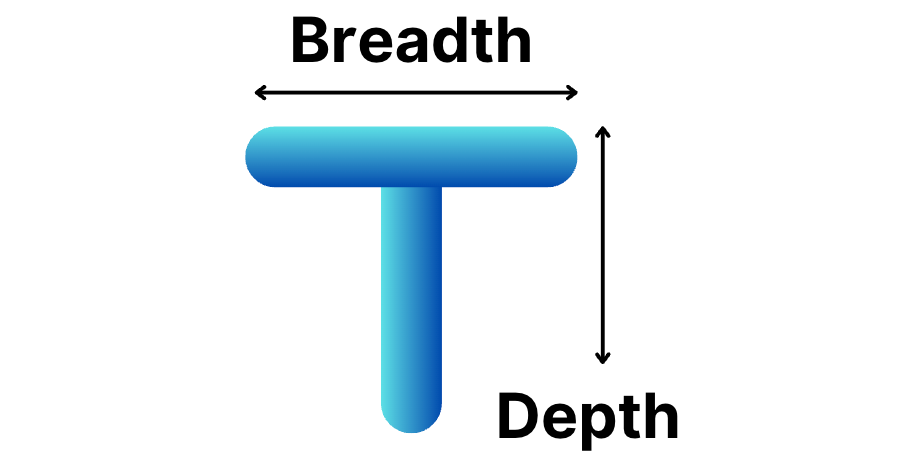
However, learning the breadth of the field and 2–3 areas to sufficient depth takes time, and the more senior you get, the more specialised you become, which makes acquiring these T-shaped skills difficult.
The best way to learn anything is through practice, so take your time and explore all your learning opportunities when you are a relatively junior data scientist.
Careers are long and span decades. Don’t rush to become a staff or principal-level data scientist before you are 30; there is no need. Enjoy the journey and absorb as much knowledge as you can.
Side note: I was listening to this podcast from the developing dev newsletter, in which a 28-year-old Google staff engineer said you wished he grew slower. So, I am not talking complete rubbish!
28 Year Old Staff Engineer @ Google
Summary & Further Thoughts
If you get these four things right early on, you set yourself up perfectly in your career. So, remember the key points are:
- Solve business problems, and stop worrying about technical solutions.
- Have a vision for your future career development and regular check-ins to ensure this is what you want.
- Save and invest your money in something comfortable for you.
- Don’t rush through the levels; allow yourself time to learn and gain vast knowledge.
Another Thing!
I have a free newsletter, Dishing the Data, where I share weekly tips and advice as a practising data scientist. Plus, when you subscribe, you will get my FREE data science resume and short PDF version of my AI roadmap!
Dishing The Data | Egor Howell | Substack
Connect With Me!
- LinkedIn or Instagram.
- My YouTube Channel to learn technical data science and machine learning concepts!
- 👉 Book a 1:1 mentoring call
Four Career-Savers Data Scientists Should Incorporate into Their Work was originally published in Towards Data Science on Medium, where people are continuing the conversation by highlighting and responding to this story.
from Datascience in Towards Data Science on Medium https://ift.tt/SXvlwAo
via IFTTT