In Defense of Statistical Significance
We have to draw the line somewhere
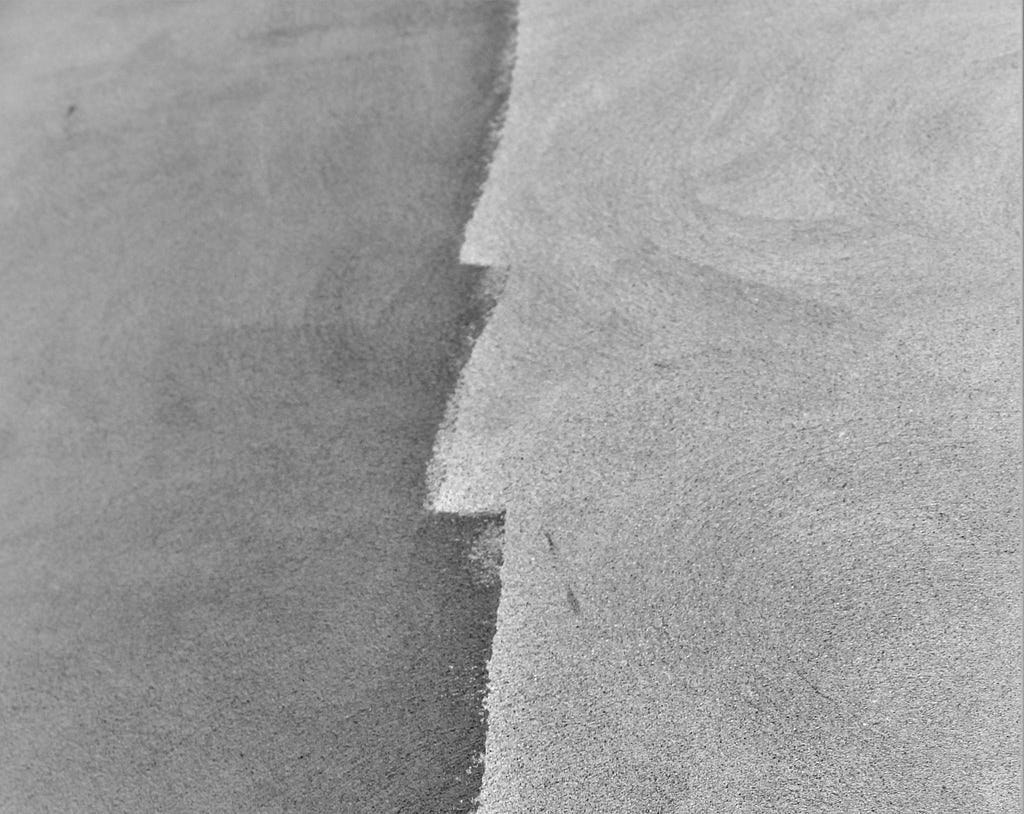
It’s become something of a meme that statistical significance is a bad standard. Several recent blogs have made the rounds, making the case that statistical significance is a “cult” or “arbitrary.” If you’d like a classic polemic (and who wouldn’t?), check out: https://www.deirdremccloskey.com/docs/jsm.pdf.
This little essay is a defense of the so-called Cult of Statistical Significance.
Statistical significance is a good enough idea, and I’ve yet to see anything fundamentally better or practical enough to use in industry.
I won’t argue that statistical significance is the perfect way to make decisions, but it is fine.
Statistical significance does not equal business significance — but so what?
A common point made by those who would besmirch the Cult is that statistical significance is not the same as business significance. They are correct, but it’s not an argument to avoid statistical significance when making decisions.
Statistical significance says, for example, that if the estimated impact of some change is 1% with a standard error of 0.25%, it is statistically significant (at the 5% level), while if the estimated impact of another change is 10% with a standard error of 6%, it is statistically insignificant (at the 5% level).
The argument goes that the 10% impact is more meaningful to the business, even if it is less precise.
Well, let’s look at this from the perspective of decision-making.
There are two cases here.
The two initiatives are separable.
If the two initiatives are separable, we should still launch the 1% with a 0.25% standard error — right? It’s a positive effect, so statistical significance does not lead us astray. We should launch the stat sig positive result.
Okay, so let’s turn to the larger effect size experiment.
Suppose the effect size was +10% with a standard error of 20%, i.e., the 95% confidence interval was roughly [-30%, +50%]. In this case, we don’t really think there’s any evidence the effect is positive, right? Despite the larger effect size, the standard error is too large to draw any meaningful conclusion.
The problem isn’t statistical significance. The problem is that we think a standard error of 6% is small enough in this case to launch the new feature based on this evidence. This example doesn’t show a problem with statistical significance as a framework. It shows we are less worried about Type 1 error than alpha = 5%.
That’s fine! We accept other alphas in our Cult, so long as they were selected before the experiment. Just use a larger alpha. For example, this is statistically significant with alpha = 10%.
The point is that there is a level of noise that we’d find unacceptable. There’s a level of noise where even if the estimated effect were +20%, we’d say, “We don’t really know what it is.”
So, we have to say how much noise is too much.
Statistical inference, like art and morality, requires us to draw the line somewhere.
The initiatives are alternatives.
Now, suppose the two initiatives are alternatives. If we do one, we can’t do the other. Which should we choose?
In this case, the problem with the above setup is that we’re testing the wrong hypothesis. We don’t just want to compare these initiatives to control. We also want to compare them to each other.
But this is also not a problem with statistical significance. It’s a problem with the hypothesis we’re testing.
We want to test whether the 9% difference in effect sizes is statistically significant, using an alpha level that makes sense for the same reason as in the previous case. There’s a level of noise at which the 9% is just spurious, and we have to set that level.
Again, we have to draw the line somewhere.
Now, let’s deal with some other common objections, and then I’ll pass out a sign-up sheet to join the Cult.
Statistical significance is arbitrary.
This objection to statistical significance is common but misses the point.
Our attitudes towards risk and ambiguity (in the Statistical Decision Theory sense) are “arbitrary” because we choose them. But there isn’t any solution to that. Preferences are a given in any decision-making problem.
Statistical significance is no more “arbitrary” than other decision-making rules, and it has the nice intuition of trading off how much noise we’ll allow versus effect size. It has a simple scalar parameter that we can adjust to prefer more or less Type 1 error relative to Type 2 error. It’s lovely.
People misinterpret frequentist p-values to indicate the probability that the effect is zero. To avoid this mistake, they should use Bayesian inference.
Sometimes, people argue that we should use Bayesian inference to make decisions because it is easier to interpret.
I’ll start by admitting that in its ideal setting, Bayesian inference has nice properties. We can take the posterior and treat it exactly like “beliefs” and make decisions based on, say, the probability the effect is positive, which is not possible with frequentist statistical significance.
Bayesian inference in practice is another animal.
Bayesian inference only gets those nice “belief”-like properties if the prior reflects the decision-maker’s actual prior beliefs. This is extremely difficult to do in practice.
If you think choosing an “alpha” that draws the line on how much noise you’ll accept is tricky, imagine having to choose a density that correctly captures your — or the decision-maker’s — beliefs… before every experiment! This is a very difficult problem.
So, the Bayesian priors selected in practice are usually chosen because they are “convenient,” “uninformative,” etc. They have little to do with actual prior beliefs.
When we’re not specifying our real prior beliefs, the posterior distribution is just some weighting of the likelihood function. Claiming that we can look at the quantiles of this so-called posterior distribution and say the parameter has a 10% chance of being less than 0 is nonsense statistically.
So, if anything, it is easier to misinterpret what we’re doing in Bayesian land than in frequentist land. It is hard for statisticians to translate their prior beliefs into a distribution. How much harder is it for whoever the actual decision-maker is on the project?
For these reasons, Bayesian inference doesn’t scale well, which is why, I think, Experimentation Platforms across the industry generally don’t use it.
The Church of Statistical Significance
The arguments against the “Cult” of Statistical Significance are, of course, a response to a real problem. There is a dangerous Cult within our Church.
The Church of Statistical Significance is quite accepting. We allow for other alpha’s besides 5%. We choose hypotheses that don’t test against zero nulls, etc.
But sometimes, our good name is tarnished by a radical element within the Church that treats anything insignificant versus a null hypothesis of 0 at the 5% level as “not real.”
These heretics believe in a cargo-cult version of statistical analysis where the statistical significance procedure (at the 5% level) determines what is true instead of just being a useful way to make decisions and weigh uncertainty.
We disavow all association with this dangerous sect, of course.
Let me know if you’d like to join the Church. I’ll sign you up for the monthly potluck.
Thanks for reading!
Zach
Connect at: https://linkedin.com/in/zlflynn
In Defense of Statistical Significance was originally published in Towards Data Science on Medium, where people are continuing the conversation by highlighting and responding to this story.
from Datascience in Towards Data Science on Medium https://ift.tt/lnOXwct
via IFTTT